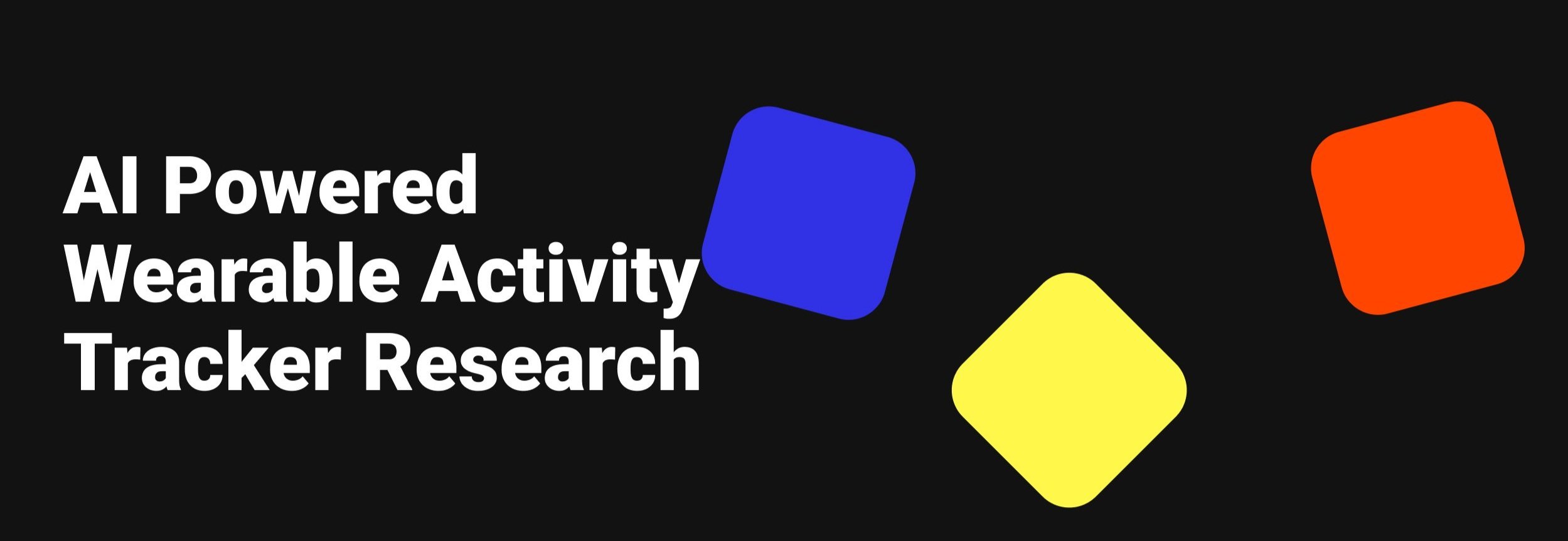
Overview
Our team conducted this research over the course of 10 weeks for the Spring 2024 User-Centered Design graduate course as part of the University of Washington's Human-Centered Design & Engineering (HCDE) program. With wearable activity trackers on the rise, an insurmountable number of family, friends, and peers are all participating in this trend. As fellow researchers and designers surrounded by this technology, we aim to critically examine the technology influencing our community members to learn how we can improve this technology and, in turn, improve the health and well-being of our communities.
My Role
Product Designer & Researcher
Time Frame
10 Weeks
Participant Pool
5 Avid smartwatch users, ages 24 - 37
Programs
Figma, ATLAS.ti Web, Survey Monkey, Zoom
Research Questions
1. How do people who use wearable activity trackers (smartwatches) use these devices to meet their health goals?
2. How receptive are people who use wearable activity trackers (smartwatches) to AI-based functionalities within these devices to meet their health goals?
Methodology
To effectively understand and improve the user experience using smartwatches, we have chosen four research methodologies that are well-suited to our project objectives. Each method selected intends to capture various aspects of user interaction while providing essential insights into daily usage, personal motivations, and cognitive processes. Here's a summary of the methods we used.
Dairy Study
Our approach with diary studies will allow us to collect ongoing, detailed observations about how participants use their smartwatch in their daily lives. This method encourages participants to record their thoughts and reactions as they interact with different existing features throughout the day.
Conducted Study with 5 people who currently use smartwatches
We monitored their use of smartwatches for 3 days.
We prompted them to submit feedback each day about how they used their smartwatch that day using a survey in SurveyMonkey. (Note: We changed the format of our diary log from multiple entries a day to one entry at the end of the day based on participant feedback. We received data from both versions.)
Interviews, Cognitive Walkthrough, and Design Probe
We conducted the interviews, cognitive walkthrough, and design probe within a one-hour session, following the diary study with the same participants from the diary study.
Cognitive Walkthrough
We conducted a cognitive walkthrough online with the same 5 people who participated in our diary study and interviews.
This method allowed us to understand users' cognitive processes and decision-making mechanisms interacting with smartwatches.
We asked participants to walk us through the app/device they used during the diary study, explaining the reasons behind their actions.
Participants were able to use their app or device to provide context when they spoke about the attitudes or motivations behind their actions.
Interviews
This method allowed us to better understand users' motivations and goals by engaging participants in in-depth discussions. Insights allowed us to understand their perceptions and receptiveness to AI within their personal use of their smartwatch.
We interviewed the same 5 people who participated in the diary study. We pulled insights gained from their diary study entries that allowed us to gain an in-depth understanding of their experiences using their smartwatch devices that we were able to pull into our interview.
Interviews were conducted online and recorded via Zoom.
Design Probe
We conducted a design probe online with the same 5 people who participated in our other methods.
This method allowed us to understand users' perceptions towards different AI-based functionalities within smartwatches.
We showed participants images of four different AI-based functionalities within smartwatches (based on our secondary research; see the appendix) and asked them about their thoughts and perceived value of these functionalities for their health goals.
Participants shared insightful feedback about their attitudes towards these functionalities within smartwatches and towards AI in general.
Data Analysis
Triangulation: We triangulated the behavioral findings from the Diary Study with the attitudinal findings from the Interview+Cognitive Walkthrough+Design Probe to better understand participants’ holistic experience with smartwatch.
Codebook: We created a codebook to arrive at an agreed-upon set of codes through which to analyze the qualitative feedback and create themes.
Thematic Analysis: By identifying findings from both the Diary Study and the Interview+Cognitive Walkthrough+Design Probe, we conducted a thematic analysis around the key themes within participants’ experiences with smartwatch and their attitudes towards artificial intelligence within smartwatch. The thematic analysis allowed us to understand themes around participants motivations for using these devices and why, how they typically used them and why, things they like about these devices or AI within these devices and why, things they dislike about these devices or AI within these devices and why, and things they would change about these devices or AI within these devices and why. We completed the thematic analysis using Atlas.ti Web in order to gain familiarity with this tool.
Inductive Insights
RQ1: How do people who use wearable activity trackers use these devices to meet their health goals?
Insight 1
Users Found value in having their exercise activities recorded
“I want all my data recorded, seeing it gives me a sense of achievement. So, I will want to keep doing it. It also helps me patch some things I forgot to track.” - Participant 2
“Before, I had to just trust the process right like there was no proof that my workouts were working…But when you have a smartwatch tracking your workouts, you at least feel like you got some kind of receipt for the workout” - Participant 3
Motivational Boost: Users experience a heightened sense of achievement from seeing their activity data, which motivates continued exercise.
Enhanced Accuracy: Recorded data helps fill memory gaps about untracked activities, ensuring a complete and accurate fitness log.
Proof of Effort: Having tangible evidence of workout efforts, like a "receipt" for exercise, validates the time and effort spent, enhancing user satisfaction.
Reliance on Objective Data: Access to precise workout data reduces reliance on subjective perception, increasing trust in the effectiveness of exercise routines.
Insight 2 :
Users experience negative emotional responses linked to smartwatch usage.
“I think my first thought would be, how many notifications am I getting? And the suggestions would be good, but like how do I turn this feature off? It becomes too much.” - Participant 4
“I don't like to check my watch during the run because I’ll feel strong emotions… I know that I’m going to be emotional looking at whether it’s good or bad.” - Participant 1
Addictive Behaviors: Users are motivated by accomplishing goals, begins to feel like a “chore.”
Anxiety: Users experience anxiety due to frequent monitoring and dependency on using their smartwatch.
Notifications Users feel overwhelmed with smartwatch notifications, some entirely avoid looking at smartwatch data due to expectations of emotional responses.
RQ2: How receptive are people who use wearable activity trackers to AI-based functionalities within these devices to meet their health goals?
Insight 3 :
Users think AI recognizing user activities and daily life is valuable to achieve their health goals.
“My body battery... it's hard to track my sleep and my average round... it could almost create those goals or plans for me based off of being on my wrist every day for 12 plus hours.” - Participant 1
“If it's not as an active thing, right. Like, if it's a busy work day, and it's not typically a gym day. I would assume then that maybe the metrics would be lowered for that day, like the goals would be lower.” - Participant 4
Personalized Goal Tracking: Users appreciate AI's ability to recognize and track their daily activities to effectively plan their goals and stay motivated.
Customizable and Realistic Goals: AI's recognition capabilities can set realistic and personalized health goals based on users' daily routines.
Flexible Scheduling: Users find value in AI's ability to identify barriers and adjust their schedules to make achieving health goals easier.
Better Health Management: Users believe that AI can provide a overall view of their health by integrating various data points, which helps them manage their health goals more effectively.
Insight 4 :
Users dislike AI “decision-making” because of control and inaccuracy
“Because at the end of the day, I don't want anybody to do anything for me. I wanna execute these tasks. I just need, you know, I need like a little bit of help...I need to be in control. So for me, this would not be valuable unless it was letting me in on the decision-making process.” - Participant 3
“(About a similar app they’ve used) I've used an app like this before...It had this function, but I think it didn't work well. It wasn't very useful. I think maybe its data wasn't very accurate. It said it would wake me up during light sleep, but I still felt very tired.” - Participant 2
Control: Some users expressed wanting to do things on their own but just needing help from AI, instead of AI doing it for them.
Wake Time: Some users feel that sleep is a sensitive, personal thing and that they would prefer to determine their own wake time.
Inaccuracy: Some users expressed concern over what data would be used to wake them up, if it is accurate, and that they’d be frustrated if it isn’t accurate.
Reflection
Generally I learned more about users’ experiences with smartwatches for their health goals. I learned that users value some affordances for their health goals (e.g., tracking their activities) but that these smartwatches can also create other challenges (e.g., anxiety). Also, some insights into AI-based functionalities which users are more receptive to (e.g., recognizing data, recommendations) and some AI-based functionalities that users may be less receptive to (e.g., predicting, decision-making). Practically speaking, I also all gained more experience with using Atlas.ti Web for qualitative analysis.
If we were to do this again, it might be useful to have more backup participants because there were a number of participants that dropped throughout the study. If we had more time, it might’ve also been useful to look more into the photos to assess the environments/context in which users typically use their smartwatches